Campaign Optimization
Optimizing your campaign after launch is crucial for improving ROI, reaching your target audience, and experimenting with different approaches.
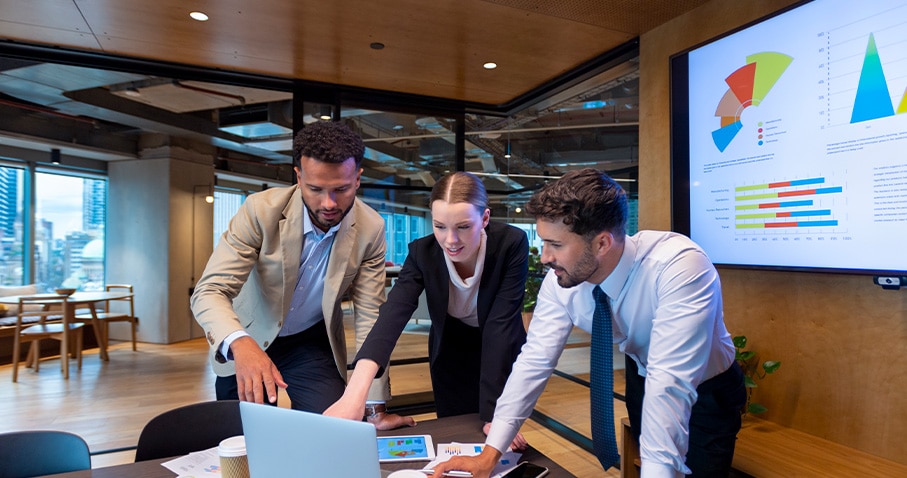
- Test and Learn Execution
- Evaluation and Optimization
“Test and learn” campaigns leverage a sprint-based approach to set up, experiment and iterate based on results.
The “test and learn” strategy is designed to drive improvement through the funnel by driving increased conversion in both lead generation and sales acceleration.
Experimentation is key to agile marketing as it enables teams to quickly understand and adapt to customer behavior.
-
What?
- The process of discovering, validating, and delivering value to customers
- Designing and running experiments to test assumptions about consumer behavior and help develop solutions that are based on conclusive outcomes
-
Why?
- To develop relevant value propositions and solutions based on a deep understanding of what motivates our target customers and use the learnings to deliver value to the customer
-
When?
- Testing and developing solutions should take place once the market environment and target customers are studied and understood
-
How?
- By operating in a highly collaborative team environment combining CX practices, advanced analytics, and Agile way of working
- Leveraging tools, such as Jira, for collaborating and tracking experiments
- Sharing and leveraging insights from previous use cases
Experimentation starts with a hypothesis you want to validate and concludes with definite outcomes that help you frame suitable actions and remedies.
Hypotheses are defined prior to experimentation and have standard components.
A complete hypothesis has four components. The first statement follows: “If ____, then ____, because ____.” The second statement follows, “I will track the desired result by ____,” The 1) variable, 2) desired result and 3) rationale, 4) tactic are the four elements of your hypothesis that should be researched, drafted and documented prior to building and setting an experiment live.
- Example 1:
If we test the placement and visibility of the “Contact Us” CTA on the campaign landing page, then we will see an increase of xx% of contact us forms being completed, improving our lead generation funnel. I believe this to be true because the Contact Us link isn’t a clear CTA – a stronger visual call to action with a differentiated button style is more likely to drive clicks, filling our lead funnel. I will track the desired result by running a series of A/B or multivariate tests testing the color, size and look and feel of the CTA.
- Example 2:
If we add a second, visible contact us form on the campaign landing page, then we will see an increase of xx% in contact us forms being completed, improving our lead funnel. I believe this to be true because the CTA to "contact us" on the landing page is not currently highlighted and doesn't look clickable. I will track the desired result by running a series of A/B tests to learn if having a visible form on the landing page helps conversion, and I will then test placement and look and feel of the form in further tests.
Based on the campaign objective, design experiments that run during the sprint.
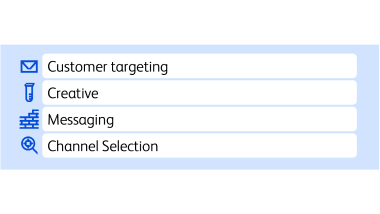
What to test?
Example of test:
- Test 1 – test brochure through LinkedIn Ad targeted at Nurse Managers
- Test 2 – test brochure through Email targeted at Nurse Managers
How to test and measure
Types of experiments:
- A/B testing
Vary one parameter between two tests and compare results
- Multivariate testing
Vary a combination of parameters between two tests and compare results
How to track impact:
- Primary success metric – KPI that indicates achievement of business objective (e.g. reach, impression for awareness campaign)
- Secondary success metric – Other metrics that support the primary success metric (e.g. CPM)
When defining marketing experiments, you should follow best practices to ensure you are able to draw valuable insights.
We recommend leveraging an experimentation template to set up hypotheses, define experiments, and track outcomes.
Step-by-Step Guide:
- Hypotheses for experimentation should be defined by the OOT core team, led by the Product Owner and Digital Marketer
- To define hypotheses, begin by indicating which variable is being tested (e.g., channels, targeting, creative)
- Next, define what hypotheses will be tested based on data points gathered from campaign inputs, indicating the metrics you are targeting and the method (e.g., we believe that the conversion rate will increase by 2% by leveraging Google Ads)
- Ensure that you indicate what channel you are targeting (Note: depending on the type of experiment, it could be multiple)
- Indicate who the target customer is (e.g., what persona are you targeting)
- Finally, indicate which stage in the funnel you are targeting based on your goals and tactics (e.g., targeting lead generation, versus targeting sales acceleration)
How to set up experimentation to drive maximum value:
Key levers that can be considered to optimize for revenue based on campaign performance:
Making decisions and optimizing campaigns based on performance:
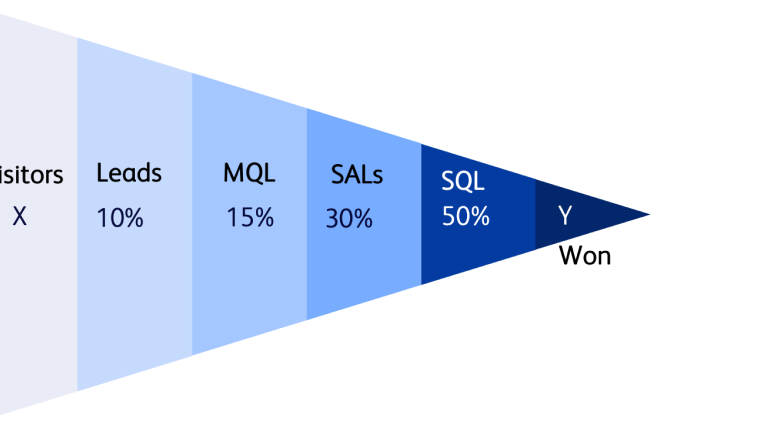
How to determine performance?
Critically examine the conversion rates in your funnel. Is there an identifiable bottleneck between steps?
What tests can be designed to address the bottleneck that the customer is encountering?
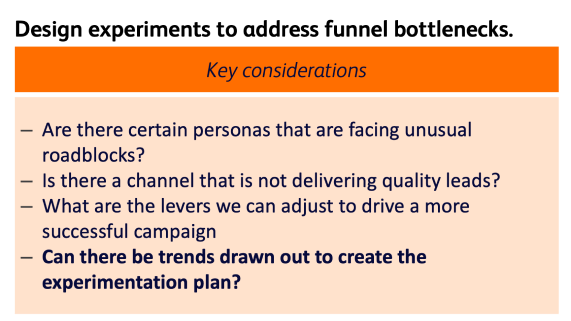
How to optimize campaigns?
How to track impact:
Primary success metric – The conversion rate lift the experiment manages to achieve